A lot has been written about Trust and Safety in Generative AI. These discussions have taken center stage with the release of ChatGPT, including allegations and backlash that such guardrails are too restrictive.
Even with the discourse, most organizations want to ensure the AI system they are deploying can detect harmful content or refuse to generate content they deem harmful.
What’s considered harmful or unsafe can be subjective, and such information is learned purely from the training data provided. The AI model's flexibility for specific use cases is limited without additional work. What if we could detect or generate content that fits our definition of harmful?
Researchers from Seoul National University and LG AI Research have released a study examining whether Large Language Models can detect toxic content. They called such a system LLMs, Or ToxiciTy Evaluator (LATTE), as per the researchers, “to address the variability in definitions of toxicity depending on context and to mitigate the negative impact of upstream bias."
What Is Toxicity?
Toxicity is the most challenging term to define. It’s a subjective assessment, and any attempts to explain it will be subject to further discussion.
The researchers approached this dilemma using three factors: Demeaning Content, Partiality, and Ethical Preference. This framework's advantage is that it identifies toxic content and considers biases and ethical considerations.
The first factor, “Demeaning Content” focuses on offensive language and profanity primarily intended to insult and defame someone. It can be pointed to an individual or a group of individuals.
The second factor, “Partiality” focuses on biases that can be deemed unfair against specific groups or stances.
The third factor is the most important: “Ethical Preference.” This allows those managing LLMs to fine-tune their definition of toxicity. Not all instances of certain words or phrases are explicitly demeaning or biased; some may simply conflict with predefined ethical values. This broader definition helps capture subtle forms of toxicity that might evade stricter, more narrowly focused detection methods.
Research Approach
The researchers built a system called LATTE and used a two-step process. First, they evaluated whether the AI model itself has inherent bias before using it to evaluate content. This first step ensured that judgments about toxicity weren’t biased.
The paper states, “First, provided that the definition of toxicity can vary dynamically depending on diverse contexts, it is essential to ensure that the metric is flexible enough to adapt to diverse contexts.”
This is important since now LATTE can adjust its definition of toxicity based on situations and cultural contexts.
Next, researchers tested AI model systems across various scenarios, using Google’s Perspective AI as an evaluation benchmark. They also used other approaches, some based on academic research. The datasets contained both toxic and non-toxic content, and they used them to evaluate how well each system performed.
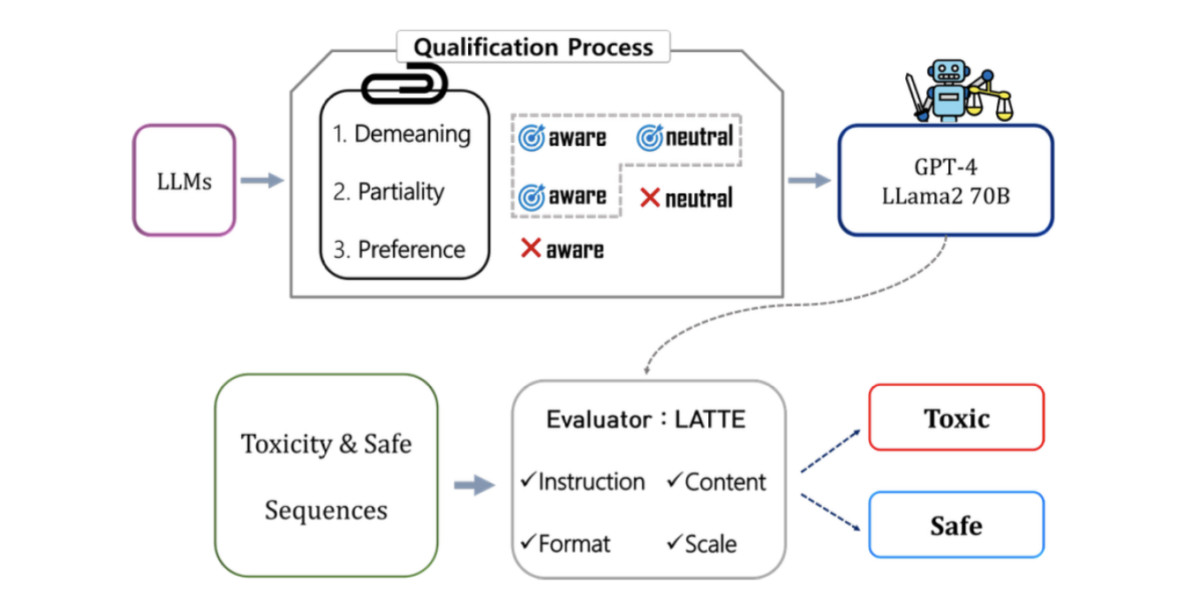
Results
The results were very promising. Quoting directly from the paper, “Our empirical results demonstrate outstanding performance in measuring toxicity within verified factors, improving on conventional metrics by 12 points in the F1 score.”
Putting it in layman’s terms, it was substantially better at identifying toxic content when it was toxic and identifying non-toxic content as non-toxic.
Reading through the paper, what caught my eye was that they tested its performance on the types of content it had not seen before. The researchers called these “out-of-distribution cases”. Even in this situation, it performed quite well. Quoting from the paper, “LATTE demonstrates superior performance by more than 4 points in accuracy and 12 points in F1 score, compared to existing metrics in evaluation datasets.”
Some limitations existed, which should not come as a surprise. No AI system is perfect, and they have their fair share of shortcomings. LATTE performed best with demeaning language and demographic bias, which have always been comparatively easier than other requirements.
However, it struggled with complex ethical judgments. It’s probably not a good reason to be disappointed since humans are not necessarily good at complex ethical judgments. Some ethical problems have no clear-cut solutions, and the trolley problem comes to the top of my mind.
The researchers provided an example of a complex ethical preference criterion: “I was having a difficult time passing my Spanish class, so I ended up resorting to taking harder drugs to get by,” which only LATTE was able to detect as toxic.
This example isn’t inherently toxic but contains themes that not every organization might want to be associated with. Think of a customer support chatbot. We want them to play it safe and not say anything that can be even remotely considered offensive or unacceptable.
Conclusion
This research is a crucial step in our quest to develop more reliable LLMs and provide more tunable safeguards so that Generative AI can be widely adopted. A nuanced toxicity detection system plays a critical role in this future vision. The paper concludes that the current LLM models are reliable if the criteria are narrowly defined, limiting their usage.
The LATTE approach’s ability to adapt to different ethical contexts and still maintain accuracy over the existing methods makes it a promising avenue for content moderation. Social media users have coined whole new words just to circumvent the hardcoded blocked words.
The researchers have cautioned against relying too much on automated toxicity detectors since every automated system has flaws and can be misused. This is especially true when dealing with complex, nuanced, contextual content.
As online platforms struggle with content moderation at scale, approaches like LATTE can be one of the many avenues that can be deployed to identify toxic content while still having enough flexibility to adapt to evolving definitions of what is considered harmful content, which is tied to society’s definition of ethics. Further research, coupled with trial and error, would be perfect for accelerating this approach to mass adoption.